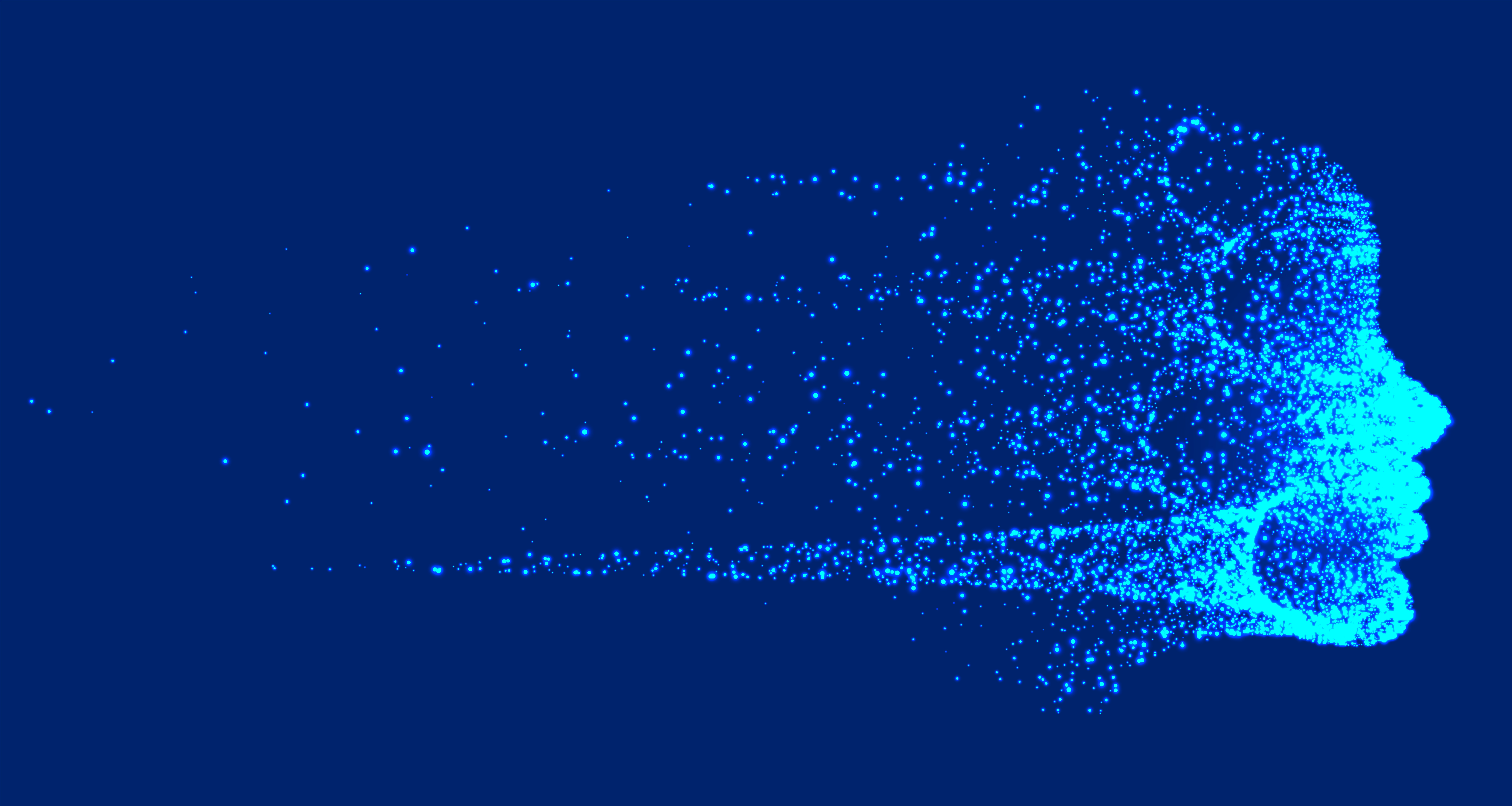
How Do Machines Learn? : Understanding Machine Learning

How Do Machines Learn?
You've probably heard by now that AI is going to save humanity. It improves your appearance in selfies, minimizes blindness, and can increase efficiency in the workplace. According to major tech companies and governments, we are currently in the "golden age" of artificial intelligence.
When people refer to "AI," they usually mean "machine learning," a more technical term for the technology in question. Despite the label, it learns primarily from human instruction. We know that machine learning seems to belong in a dystopian science fiction novel or an action movie in which machines take over the planet, and humans rarely succeed. But it is now a reality! Machine learning technology is widely used to save our planet and fight climate change. If this topic interests you, we recommend reading this article about how machine learning can be a savior for humanity.
So, let's get started describing machine learning to you.
Machine learning is a type of artificial intelligence, and a computer scientist will give you a technical answer that includes algorithms, pixels, and supervised and unsupervised learning.
If you have a basic idea about machines that can learn, let's dive into the magical world of machines together.
In particular, you will find frequently asked questions about machine learning, such as;
- What is machine learning?
- Can machines learn?
- How do machines learn?
- Some Machine Learning Solutions
Can Machines Learn?
Yes, machines are capable of learning. A computer "learns" by searching through massive amounts of data for recurring patterns; when it finds one, it modifies the program to reflect the "reality" of what it has discovered. This process is known as machine learning. The more information that the machine is exposed to, the "smarter" it becomes. And once it has seen enough patterns, it starts coming up with predictions. In contrast to people, however, computers cannot generalize their knowledge or move their learning from one application to another.
Machines are objective fact-finders and do not, contrary to what Hollywood would have you believe, hold judgments about the fate of humanity; instead, they learn "from prior calculations to create dependable, repeatable conclusions and results," as explained by SAS. They can perform calculations in minutes that would take hundreds of data scientists a year to complete. Therefore they are clearly more clever than the brightest humans.
What is Machine Learning?
Machine learning is using AI and data science to discover hidden patterns in data without explicitly defining the meaning of those patterns. Algorithms can learn from data and make predictions without being explicitly programmed using this method. Just like humans, machine learning improves with experience and exposure to new data.
Everything you ever wanted to know about machine learning, in a nutshell:
- The field of machine learning, which allows computers to acquire new skills without being explicitly taught, lies at the crossroads of computer science and statistics.
- Supervised learning and unsupervised learning are the two main types of machine learning issues. We will get to this later in this article.
- An OLS regression is a relatively straightforward example of a machine learning algorithm.
How Do Machines Learn?
In a few words: algorithms. Humans give algorithms, which are sets of rules that help computers solve problems, a lot of data so they can learn from it. In general, a machine learning algorithm gets more accurate as it gets more data.
Based on how they work with data, machine learning algorithms can be put into two main groups: supervised and unsupervised. Because of the different ways they analyze data, these two types of machine learning are better at solving different kinds of problems. For all kinds of machine learning to work, algorithms need a lot of data to learn from.
Supervised Learning
In supervised learning, systems are taught to perform a job by being shown examples of how to perform it, both in terms of input and output. Using example input-output pairs, supervised learning in machine learning translates input to its outputs. This method of learning draws conclusions about an unknown function based on sets of training examples that have been labeled and categorized. Training datasets for supervised learning often include metadata rather than image inputs and are labeled along the X and Y axes.
The University of California, Irvine Iris Dataset is an often-cited instance of a supervised learning dataset in which the inputs are various variables representing classes of irises, and the predicted attribute is the class of iris (petal width and length, sepal width and length).
During the training phase, a supervised learning model is asked to generate predictions and then adjusts its behavior based on the results of those predictions. This iterative process of making educated guesses and verifying them with new data is repeated until the model satisfies some criterion for accuracy on the provided training data. Both logistic regression and back propagation neural networks are utilized as supervised learning algorithms. It's common for classification and regression to be at the heart of supervised learning issues in machine learning.
Unsupervised Learning
While training a machine learning model, the designer of the model is not required to "supervise" it using unsupervised learning. In unsupervised learning, the model is not dependent on a meticulously ordered and labeled data set in order to make discoveries. This is why unsupervised learning is often used to train machine learning models, as it allows for the usage of datasets that have not been labeled.
When it comes to unsupervised learning, algorithms are free to discover patterns in data without being guided by labels or names for input variables. Unsupervised learning models make predictions for both the inputs and the outputs, in contrast to supervised learning models, which are only given the outputs to predict. This is achieved by identifying salient patterns among the inputs and linking those to attainable outcomes.
Examples of unsupervised machine learning model-specific algorithms are K-means and the Apriori algorithm. Clustering, dimensionality reduction, and learning associations are all common machine learning tasks that benefit from unsupervised learning.
Cameralyze Machine Learning Solutions
The adoption of machine learning has reduced the time it takes for businesses to gain insights, which has resulted in greater returns on investment. That's why ML is essential for any business.
Cameralyze provides over a thousand machine learning-based solutions, including facial recognition, object recognition, emotion detection, and more, to help you implement this complex and intelligent technology into your business and make it more efficient and autonomous.
Let's take a quick look at the machine learning solutions Cameralzye provides:
Face Recognition Solution
The ML-Based Face Recognition Solution provides a one-of-a-kind encounter by detecting, tracking, identifying, and analyzing faces with the highest accuracy and performance, all without the need for any coding.
Cameralyze Facial Recognition System allows for unmatched protection, efficiency, and safety.
Facial Emotion Recognition
Using machine learning, Cameralyze provides the most precise and automated approach for identifying human emotions from facial expressions, including the universal expressions of happiness, sadness, anger, surprise, fear, calmness, and confusion.
Human Detection Solution
Using Cameralyze's real-time human recognition solutions in machine learning technologies, you can quickly and accurately identify individuals in any photo, video, or live stream. With this system, monitoring tasks may be performed remotely, which reduces overhead and boosts morale among employees.
Demographic Analysis
Using machine learning methods, it can determine the age and gender of people in any photo, movie, or live broadcast.
You can acquire an understanding of the demographics of your current and prospective customers and customize your service to meet the specific needs of each customer. It will help you to understand market demand better.
With Cameralyze, you can track your site's visitors, personalize your content for each user based on their profile information, and reach your intended audience better.
Final Words
Machine learning has shortened the time it takes for businesses to get insights, leading to higher ROI. Because of this, ML is a must-have for any business. For a deeper dive into the practical benefits and applications of machine learning, we suggest reading our other articles on the topic.
To apply this complicated and intelligent technology to your business to make it efficient and autonomous, Cameralyze offers more than a thousand machine learning-based solutions such as face recognition, object recognition, emotion detection, and so on.
Thanks to its easy-to-use interface and no-code platform, you do not have to learn all this hard stuff to apply machine learning to your business. Cameralyze will handle the hard work for you! Start a free trial now!
Learn more about Machine Learning:
- What Is Precision and Recall in Machine Learning?
- The Future of Emotion Recognition in Machine Learning and AI
- Top 5 Interesting Machine Learning Stats in 2022
.png)