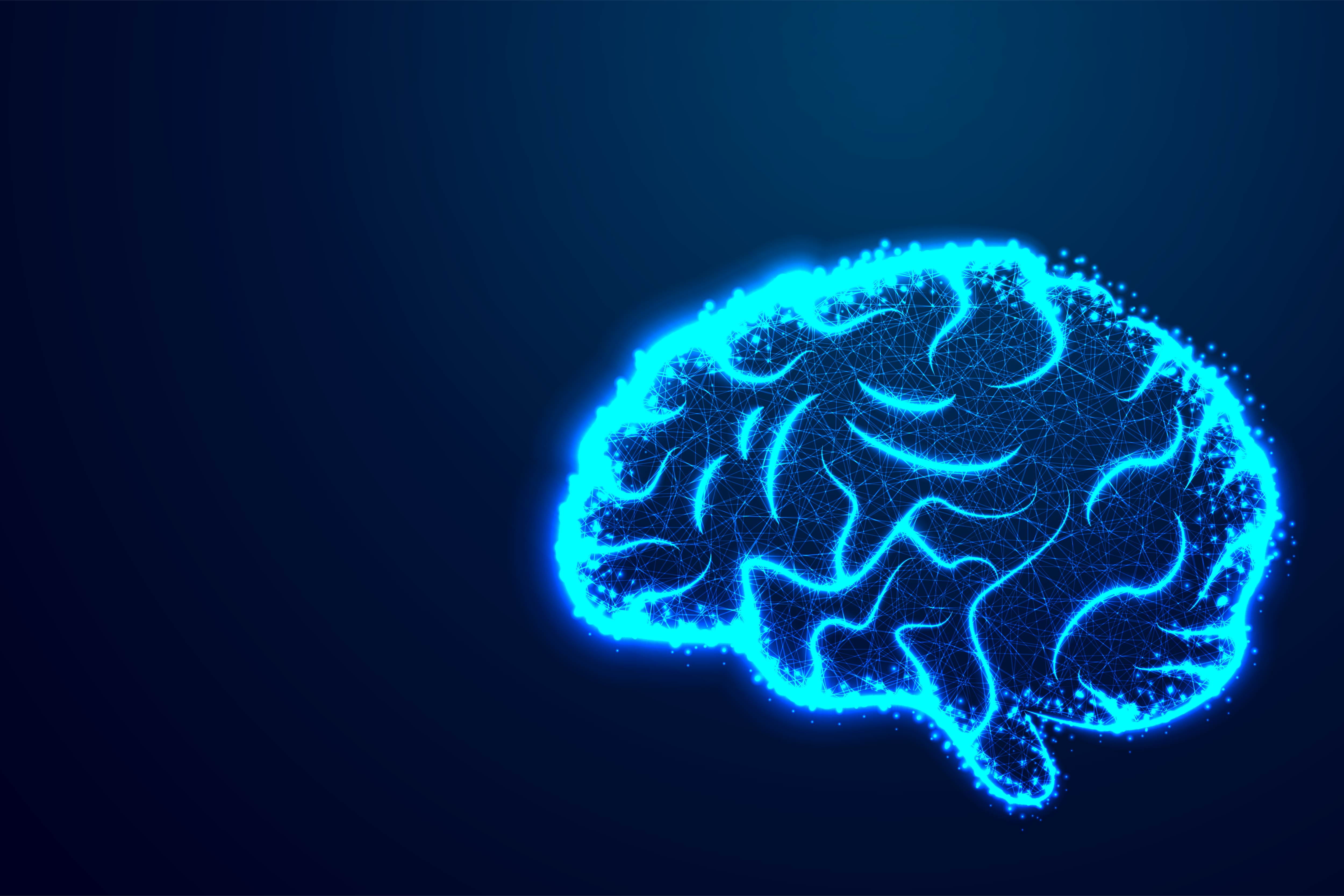
Image Annotation: Definition, Types & Image Annotation Solution

What is Image Annotation?
Computer vision is the field of study that enables computers to acquire human-level visual perception and comprehension from digital photos and videos, and image annotation plays a crucial part in this. Self-driving automobiles, tumor detection, and autonomous drones are just a few of the incredible artificial intelligence (AI) applications made possible by advancements in computer vision technology. However, without image annotation, most of these impressive uses of computer vision would not be conceivable. Annotation, often known as picture tagging, is a crucial first step in developing the vast majority of computer vision models. Datasets are essential for machine learning and image recognition to succeed with deep learning approaches.
This blog article will answer frequently asked questions such as;
- What Is Image Annotation?
- How Does Image Annotation Work?
- What Are The Types Of Image Annotation?
- Image Annotation Solutions
- How Long Does Image Annotation Take?
- How to Find Quality Image Data?
The Definition of Image Annotation
Annotating a picture using text labels, automated annotation tools, or both is called "image annotation" in machine learning and deep learning. It is used to demonstrate the data properties you want your model to identify automatically. Adding metadata to a dataset is what happens when you annotate a picture.
Annotating images can also be referred to as "tagging," "transcribing," or "processing," and is a kind of data labeling. Continuous, stream-like, or frame-by-frame annotating of videos is also possible.
Annotating images with labels indicating which characteristics should be recognized by the machine learning system allows you to use such images for supervised learning training. Following deployment, you'd want your model to be able to identify those traits in unlabeled photos and behave accordingly.
The most popular applications of image annotation include object recognition, boundary detection, and segmentation for the purpose of gaining context, meaning, or an overall picture of an image's contents. Training, validating, and testing a machine learning model to get the intended output requires a large quantity of data for each of these applications.
How Does Image Annotation Work?
Any free or open-source data annotation tool will do the work for annotating images.
The most widely used open-source program to annotate images is the Computer Vision Annotation Tool (CVAT). Images will need to be annotated by a professional due to the complexity of the task and the sheer volume of data involved. Organizations can have their own data scientists classify pictures. Still, for more complicated, in-depth projects, it is generally necessary to contract with an external artificial intelligence (AI) video annotation service provider.
Single-frame or multi-frame annotation is made easy with the help of the annotation tools' various feature sets. The objects in a picture are labeled using one of the annotation methods described below; the number of labels per image might vary widely depending on the scenario.
Types of Image Annotation
Object identification, position estimation, key-point detection, image classification, object detection, object recognition, image segmentation, machine learning, and computer vision models all make use of picture annotation.
Image Classification
Image classification is the most straightforward and quick method of image annotation since it assigns a single tag to each picture.
Classifying a set of photos of supermarket shelves to determine whether or not any of them contain soda is one such example. If you want to capture abstract information like the time of day based on whether or not automobiles are present in a photo, or you want to filter out pictures that don't satisfy the criteria from the get-go, this is the way for you. However, although classification is the quickest image annotation in terms of providing a single, high-level label, it is also the most imprecise since it does not specify where the item is inside the picture.
Also Read: One Of The Ai Technologies: Image Classification
Object Detection and Object Recognition
Picture categorization is expanded upon by object detection or identification models, which can determine the presence, position, and number of things in an image.
In order to identify the precise location and quantity of objects in an image, we had to create borders around all of the recognized ones during the image annotation process for this type of model. The primary distinction is in the fact that sub-classes are identified inside images rather than the whole picture being assigned to a single category.
Instead of treating the whole picture as a single class, as is done in traditional image classification, the class location is an additional parameter. Bounding boxes and polygons are two examples of labels that can be used to annotate objects in a picture. Person detection is widespread use of object detection. To detect people in images, the computer must constantly examine them for distinctive characteristics. By monitoring the evolution of features over time, anomalies can be identified via object detection applications.
We suggest the following readings for anyone interested in object detection and object recognition:
Image Segmentation
Image segmentation is a technique for simplifying digital images by separating them into smaller parts called "segments." This allows for more precise processing and analysis of individual components of the image. Segmentation, in a technical sense, is the process of labeling pixels in order to locate specific objects, people, or other features of interest in a given picture.
Boundary Recognition
The term "boundary detection" refers to the method used to identify and define the locations of appropriate boundaries between items in a scene.
Boundaries can be the edges of a particular object or parts of the image's topography. Once an image has been appropriately annotated, it can be used to find patterns in other images that haven't been annotated. Autonomous cars need to be able to recognize boundaries in order to drive safely.
How Long Does Image Annotation Take?
Annotation times depend significantly on how much data is needed and how complicated the annotation goes with it. Even companies that do image annotation have a hard time figuring out how long it takes before they have to label some samples and use the results to make an estimation. Annotations that work on a small number of simple objects are faster than annotations that operate on objects from thousands of categories. In the same way, annotations that only need the image to be tagged take much less time to finish than annotations that need to point out multiple key points and objects.
But even then, there is no guarantee that the consistency and quality of the annotations will make it possible to make accurate estimates.
Even though automated image annotation and semi-automated tools help speed up the process, a human is still needed to make sure the quality is always the same. In comparison to region-based objects with more control points, simple objects with fewer control points often take much less time to annotate.
How to Find Quality Image Data?
It's challenging to find reliable sources of annotated data. If there is a lack of publicly accessible data, annotations must be constructed from raw obtained data. This often comprises a battery of tests to eliminate the risk of error or contamination in the final product of the processing.
Variables like these affect how sharp a picture turns out to be:
Better results will be achieved with a larger sample size of annotated photographs. The greater your dataset is, the more varied the settings and scenarios that may be utilized for training will be.
Distribution of annotated images: Having all of your pictures in the same class isn't ideal since it reduces the diversity of your data collection and, by extension, its usefulness. You'll need a large sample size for each class to successfully train a model that excels in every setting.
Knowledgeable annotators with few mistakes may generate high-quality annotations, but your results will suffer if your annotators are all the same. There may be regional differences in vocabulary or traditions, so having numerous annotators provides redundancy and helps maintain uniformity across various groups or nations.
Business Image Annotation Solution
Cameralyze, a computer vision platform, has an integrated CVAT-based image annotation environment. Cameralyze is browser-based, cloud-native software. Cameralyze is a comprehensive tool for professional teams that allows them to annotate images and videos. Collaborative video data collection, picture annotation, AI model training and management, application development without coding, and management of large-scale computer vision systems are all possible with this tool.
Cameralyze speeds up the entire application lifecycle using automated no-code tools to automate and help accelerate monotonous integration tasks.
Image Annotation with Cameralyze
Image annotation is the process of adding data labels to an image. Typically, the annotating task requires human labor with computer assistance. For the purpose of training computer vision models, picture annotation tools like the widely used Computer Vision Annotation Tool (CVAT) are invaluable.
Have a look at Cameralyze if you are looking for a professional image annotation system that offers enterprise-level features and automated infrastructure.
Find out more about how our annotation solutions can help you with your image annotation projects, or get in touch with us.
.png)