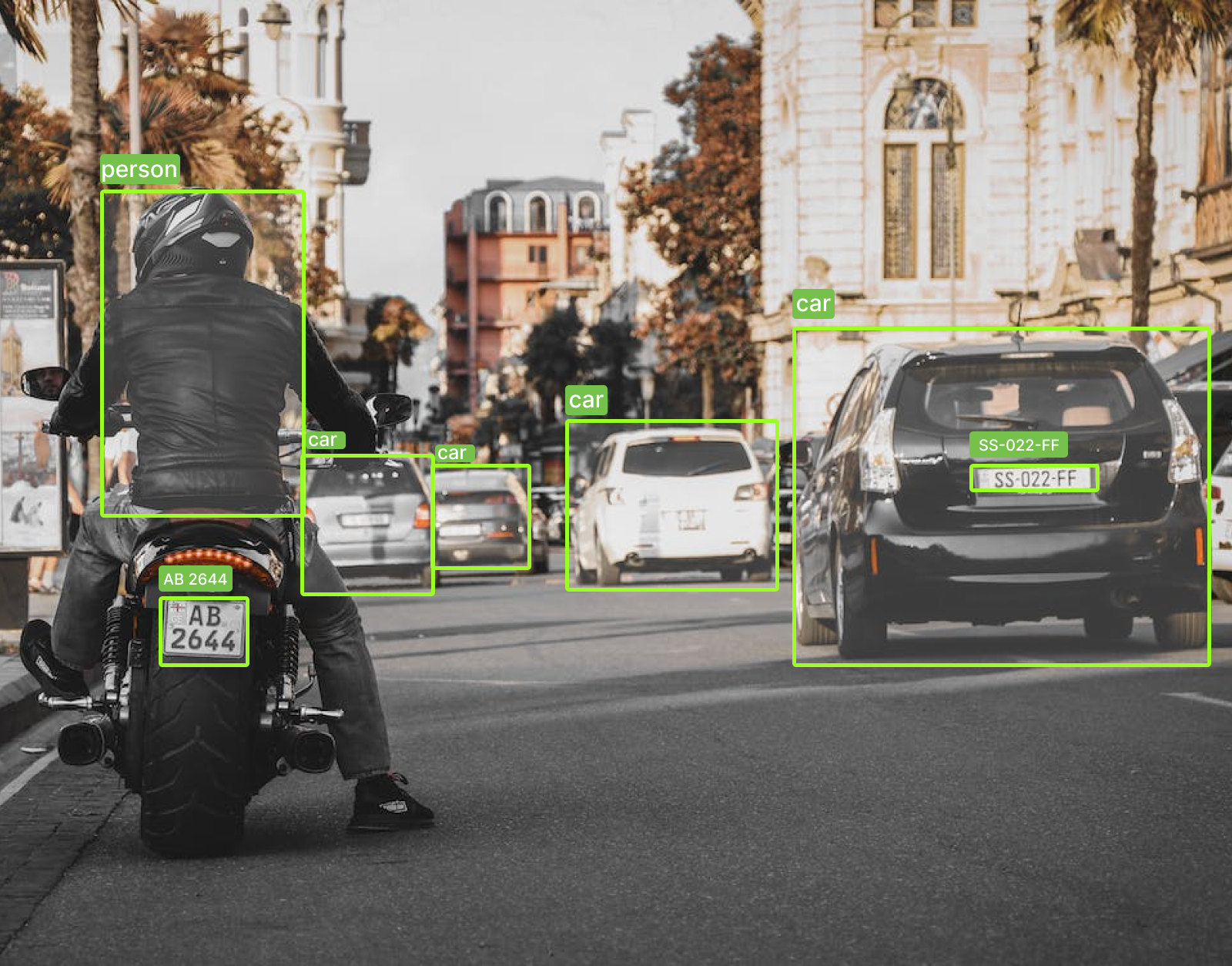
Key Challenges For Image Annotation in ML
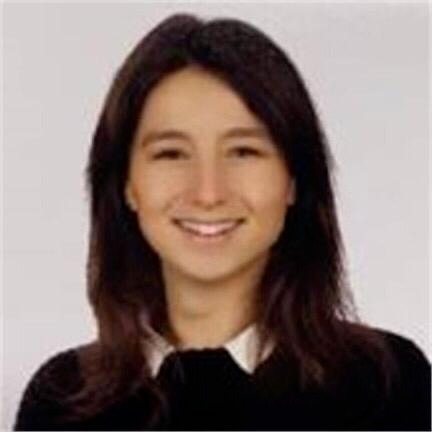
Key Challenges For Image Annotation in ML
Image annotation is a significant player in computer vision, which is the technology that allows computers to obtain a perfect understanding of digital images or videos and to see and interpret visual information in the same way as humans do.
Computer vision technology enables incredible AI applications such as self-driving cars, tumor detection, and uncrewed aerial vehicles. However, most of these remarkable computer vision applications would not be possible without image annotation. Annotation, also known as image tagging, is a crucial step in developing most computer vision models. Using deep learning techniques, data must be helpful to components of machine learning and image recognition.
This article will specifically address:
- What is Image Annotation
- Types of Image Annotation
- 4 Real Life Use Cases for Image Annotation
- Challenges For Image Annotation
Before delving further into the topic: If you need computer vision solutions for business applications, check out Cameralyze, the leading no-code computer vision platform. The platform allows you to annotate images, visualize programming, and automated deployment in one unified solution.
.png)
What is Image Annotation?
The process of labeling images in a dataset in order to train a machine learning model is known as an image annotation. As a result, image annotation is used to label the features that your system needs to recognize.
The annotation task is typically performed manually with the assistance of a computer. A Machine Learning engineer determines the labels, referred to as "classes," and feeds the image-specific information to the computer vision model. After the model has been trained and deployed, it estimates and recognizes those predetermined features in newly annotated images.
Types of Image Annotation
Annotating images is commonly used for image recognition, pose estimation, key point detection, image classification, object detection, object recognition, image segmentation, machine learning, and computer vision models. It is a technique for creating reliable datasets for models to train on, and it is thus helpful for supervised and semi-supervised machine learning models.
Different image annotation techniques are required to develop practical datasets for various purposes such as object recognition, image segmentation, etc.
1. Object Detection and Object Recognition
Object recognition and detection models go beyond image classification to determine the presence, location, and the number of objects in an image. The image annotation process for this model required drawing boundaries around every detected object in each Image, allowing us to determine the exact position and number of objects present in an image. As a result, the primary distinction is that classes are detected within an image rather than the entire Image being classified as a single class of image recognition.
Class position is an additional parameter to the class; on the other hand, in image classification, the position of the class within the Image is not essential since the entire Image is defined as a single class.
Objects can be annotated within an image by placing bounding shapes and using labels, such as people, animals, and tables. One of the most popular examples of object detection is human detection. It requires the computing device to constantly analyze frames to identify certain object properties and recognize existing objects as persons. Object detection can also be used to detect any anomalies by monitoring the change in properties over some time.
2. Image Classification
Image classification is a type of machine learning model that requires images to have a single label to identify the entire Image. The image annotation process for image classification models aims to detect the presence of similar objects in images from the dataset.
The purpose of this feature is to train an AI model to recognize an object in an unlabeled image that resembles classes in annotated images used to train the model. Tagging is another term for training images for image classification. Image classification seeks to simply identify the presence of a specific object and name it according to a predefined class.
3. Image Segmentation
The image type that allows the division of an image into multiple parts is called image segmentation. Image segmentation is used to find objects and constrained shapes in images. It is performed at the pixel level by assigning each pixel within an existing image to a specific object or class.
4 Real-Life Use Cases of Image Annotation
.png)
1. Autonomous driving
Image annotation aids in the creation of large datasets used to train autonomous driving software for self-driving vehicles.
- Monitoring of in-cabin behavior in advanced driver-assistance systems (ADAS)
- Navigation response steering
- Detection of on-road objects and dimensions
- Monitoring of movement
- LiDAR detection
2. Security and surveillance
Image annotation is a critical component of agile security. Crowd detection, night vision even in the darkest of hours, thermal vision, traffic motion, face identification for theft detection, pedestrian tracking, and other processes are aided by it. ML engineers train datasets for high-tech video equipment based on annotated images to provide round-the-clock security surveillance for a safer environment.
3. Insurance
Despite popular belief, insurance is one of the industries that will benefit the most from built-in AI. The bottom line is that AI must be trained for extreme accuracy in order to replace manual damage evaluation, which is only possible with large amounts of annotated data containing vehicle defects. Beyond the advanced assessment levels, the ML model can also predict whether or not the part needs to be replaced, and more advanced models can calculate the exact cost of the replaced part.
Help with extensive pattern recognition. Insurance will reduce response time significantly, improve customer experience, and save financial and human resources.
4. Medical Imaging
Machine learning model training is a boon to the medical industry. The modern healthcare system's success is attributed to the rapid advancement of AI. Diseases such as brain tumors, blood clotting, and a variety of neurological disorders are detected using CT scans and MRI, both of which rely on well-trained ML models and large amounts of medical image data.
Other medical image annotation use cases range from quantitative analysis for cancer cell detection to kidney stones, teeth segmentation, eye cell analysis, and microscopic cell analysis at the nanoscale. The ML model uses these datasets to perform deep learning in order to create an automated diagnosis mechanism for the healthcare industry.
Now that we've covered Image Annotation in-depth let's take a look at Image Annotation challenges.
List of Challenges For Image Annotation
Large-scale training Data: A massive amount of training data is required to develop computer vision-based visual perception AI models. Creating such massive datasets on a large scale is already a difficult task. The model will predict accurately regardless of how much training data is used to develop it.
Accuracy and Quality: The second and most significant challenge is annotation quality, as any compromise in quality or inaccurate annotation can affect the model prediction. As a result, quality is checked at several levels to ensure that each training data fed into the algorithm is unique and of the highest quality.
Annotation Platform: There are numerous image annotation platforms and tools available, each with unique capabilities for various types of annotations. The abundance of options can make it difficult to select the best tools for each project, and it can also be difficult to select the right tool to match your workforce's skill set. At this point, Cameralyze comes into play, providing a market-leading tool in the field of computer vision, and you can use the platform with minimal technical knowledge.
The final challenge is the security and privacy of datasets obtained for annotation in the form of images and videos. Yes, data annotation companies face such challenges because they adhere to data security standards or regulations in order to process the client's data in a highly secure manner.
Key Thoughts
To meet all these challenges, Cameralyze has designed and developed its platform to get the best quality results for users. However, he has created a quality tool that can easily cope with all such challenges and can add a large amount of annotation to any image or video while ensuring the highest level of accuracy. In addition, data security standards are at the highest level to ensure the confidentiality and security of data at every stage of data processing. Try the Image Annotation now.